Image cleanup and preprocessing are the sides of capture that often get ignored, yet they are central to effective data location and extraction. Even in the age of machine learning and smart capture solutions, without optimized images, the best digital transformations can still fail to provide the accurate data your business systems require.
Image cleanup and preprocessing are analogous to tuning your guitar before you play it or adjusting your sound system before you record so that the treble or bass or effects processing are adjusted for optimal sound quality. Initial image quality control and cleanup ensures that the image is as good as it can be even prior to any automated preprocessing.
Preprocessing consists of a set of steps used to produce a cleaned-up version of a document image. Preprocessing transforms the document image into a usable format for the next stages of recognition. One of the exciting aspects of preprocessing is that today, capture with machine learning can do image cleanup automatically that was never available before with improved results over time. For example, Parascript Artificial Intelligence software can preprocess poor quality document images correcting for noise and other challenges (which regular OCR engines fail miserably at), and achieve reliable data results.
Garbage In, Garbage Out (GIGO)
So, what are the problems that organizations continue to face with their document images? Capture and recognition results still require a certain level of initial quality, no matter how advanced automated preprocessing becomes. A good rule of thumb is that if a person cannot read it, neither will the machine software. Attention to document image quality preservation helps ensure reliable data results and helps eliminate GIGO.
Back to Basics: Tips to Improve Quality
We thought we’d share some recommendations for maintaining your document image quality gained through our years of industry experience:
- Understand Your Documents. Your documents may be completely unstructured, semi-structured or structured. They may have logos, tables and illustrations, paragraphs with rotated text, handwritten fields, machine-print or both. Advanced capture powered by machine learning can handle the simplest to the most complex documents. However, you can identify and correct for the difficulties that you are most likely to face with certain types of documents by knowing about their patterns: their quality features and specific challenges. For example, maybe your staff has been seeing excessive document skew on scanned images, document pages that overlap or piggyback so only part of the information is available and erroneously combined on one page. The images tend to be too dark, too light or have excessive spot noise, just to name a few common problems.
- Tailor Your Document Processing Workflow. Once you know your documents, re-examining the document processing workflow and its automation is critical. This will allow you to analyze any cleanup tasks that you might need to add to your workflow either for all of your document processing or just a subset, which typically leads to significant improvements in data results. For example, making sure that scanning equipment is regularly maintained so that scanned images don’t degrade over time. Ensuring that the staff is trained appropriately so that scanned documents are legible to the human eye. If images are out of focus or excessively skewed, etc., they have to be re-scanned before they are submitted to be automatically preprocessed.
- Work with Your Images. Organizations often have less control over the quality of their document images than they would like, but putting in place quality evaluation processes is important. Sometimes, this image quality is government regulated such as with checks in the banking industry. “Image Replacement Documents” or “IRDs” and actual check images can replace hard copy checks when they meet certain image quality criteria. Software such as CheckUsability provides organizations with the ability to automatically validate and accept or reject document images based on quality criteria that evaluates potential problem areas such as: document framing, image size, image skew, piggybacking (overlapping with another document), excessive spot noise, image focus or only a partial image.
Quality Images In, Quality Data Out
Getting back to the basics of image cleanup can appear a rather dull and time-consuming support task, but it can make all the difference by making it possible to deliver those quality data results that your business needs to solve bigger issues and deliver real value. Preprocessing for those images that can’t be cleaned up or re-scanned or re-transmitted is always a viable option considering that capture powered by machine learning is getting better and better at extracting data from our hard to read and complex documents.
If this article interested you, you may also find the latest best practices white paper useful to you…
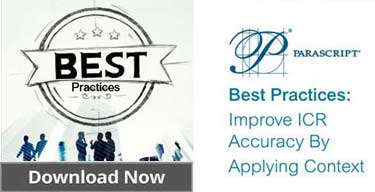